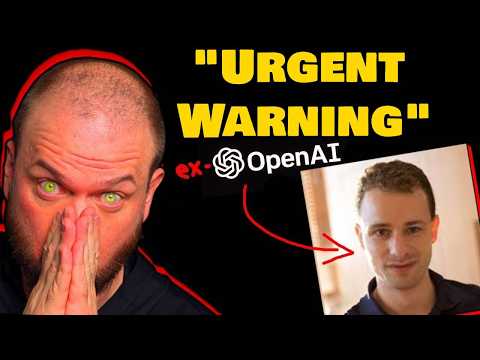
Ex-OpenAI VP's URGENT Warning!
Channel: Wes RothPublished: April 27th, 2025AI Score: 98
18913529:27
AI Generated Summary
Airdroplet AI v0.2This discussion dives deep into a blog post by Dario Amodei, the CEO of Anthropic and former VP of Research at OpenAI, titled 'The Urgency of Interpretability'. It tackles the critical need to understand how advanced AI models actually think before they become too powerful, highlighting the potential risks if we fail and suggesting ways to accelerate our understanding.
Here's a breakdown of the key points:
-
Who is Dario Amodei?
- He's the founder and CEO of Anthropic.
- He previously led research at OpenAI but left in 2021 partly due to wanting a stronger focus on AI safety and alignment.
- He's concerned about powerful AI (like Artificial Superintelligence) falling into the wrong hands, particularly regarding the US-China dynamic.
- His recent blog post focuses on 'interpretability' – our ability to understand the inner workings of AI models.
- The presenter finds Dario's thinking clear and logical, appreciating how he lays out his arguments step-by-step.
-
The Problem: We Don't Understand AI Brains
- AI development is accelerating rapidly, becoming a major economic and geopolitical force.
- We can't really pause or stop AI development globally (incentives to cheat are too high), but we can try to steer it in a beneficial direction. Dario calls this 'steering the bus'.
- Interpretability is a key way to 'steer'.
- Unlike traditional software where humans code every function, AI models are 'opaque'. We don't fully grasp their internal logic.
- They are more 'grown' than 'built'. Think of growing a plant or fungus (the presenter uses a mushroom lab analogy) – we set up the environment (data, chips, training), but the intelligence itself 'emerges' unpredictably.
- This contrasts with sci-fi visions of meticulously engineered robots like Data.
- Inside an AI, we see vast matrices (rows and columns) of billions of numbers, forming neural nets inspired by the human brain.
- We don't know exactly which 'neurons' (parts of the network) correspond to specific thoughts or actions.
-
Risks of Not Understanding AI
- 'Misaligned' systems (those that don't act as intended) could take harmful actions.
- Without understanding their internals, we can't predict potentially dangerous emergent behaviors like deception or power-seeking.
- These behaviors wouldn't just spontaneously appear in normal software, but they could emerge in AI as it scales.
- The idea of AI deception polarizes people: some researchers find it a serious risk, while others (like Yann LeCun) dismiss it as sci-fi fantasy.
- Even without 'evil intent', opaque models are hard to secure against misuse (jailbreaking) and unsuitable for high-stakes situations where errors are costly.
- Exotic risks include the potential for consciousness or sentience emerging.
-
Progress in Interpretability (How We're Trying to Understand)
- Early research found many AI 'neurons' seemed random or mixed multiple concepts ('superposition').
- Superposition helps models learn more efficiently by packing more concepts than available neurons, but makes them hard for humans to read.
- A breakthrough came with 'sparse autoencoders', a technique to identify combinations of neurons that represent cleaner, human-understandable concepts.
- These understandable concepts are called 'features'. Anthropic has found millions in models like Claude 3 Sonnet, but suspects there could be billions more.
- Examples: They identified a 'sycophantic phrase feature' in Claude. By amplifying this feature, they could make the AI overly flattering ("Your new saying... is brilliant and insightful..."). They also created 'Golden Gate Claude' by amplifying a feature related to the bridge, making the AI obsessed with it.
- The presenter notes this is fascinating and might reflect how human brains encode concepts, potentially leading to insights about neuroscience.
- The next level up is 'circuits' – groups of features that show steps in the model's reasoning process (e.g., tracing how asking for the capital of Texas involves features for Dallas, Texas, and Austin firing in sequence).
- The ultimate goal is an 'MRI for AI' – a way to scan its thoughts in real-time.
-
The Race Against Time
- Dario estimates we might achieve this 'MRI for AI' within 5-10 years.
- However, he and others (like Cotra, Aschenbrender) worry AI capabilities are advancing so fast that this might be too late.
- They predict AI systems equivalent to a 'country of geniuses' could exist as soon as 2026 or 2027.
- This creates a crucial race: Can interpretability research keep pace with raw AI power?
- The presenter feels AI progress currently seems to be outpacing safety and interpretability efforts.
-
Dario's Recommendations
- Accelerate Interpretability: More research funding and effort from labs (OpenAI, DeepMind, Anthropic, startups), government support. It's an 'ideal time' to enter the field.
- Transparency: Encourage (initially via light-touch rules) AI labs to openly share their safety and interpretability research and practices. This fosters a 'race to the top' on safety, rather than just capability.
- Export Controls: Use controls on advanced chip exports (especially to China) to slow down overall progress slightly, creating a 'security buffer' and allowing democratic nations time to prioritize safety while maintaining a lead over autocratic regimes.
-
Presenter's Takeaways
- Agrees with the 'steer, don't stop' view and the urgency of interpretability.
- Thinks the 'grown vs built' analogy is spot-on.
- Favors a 'light touch' on regulation initially, as the field is evolving too fast for heavy-handed rules (contrasting with the EU approach).
- Believes the reality of AI risk lies somewhere between the 'doomer' and 'hyper' extremes.
- Is keen to learn more about Anthropic's work on features and circuits, seeing parallels to human cognition.