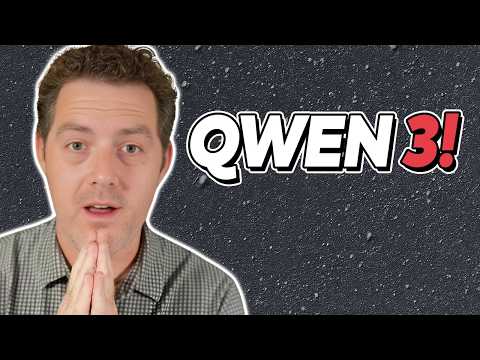
Qwen3 is a fantastic open-source model
AI Generated Summary
Airdroplet AI v0.2A new family of open-source AI models called Qwen3 just dropped, and it's seriously impressive, even giving models like Google's Gemini 2.5 Pro a run for their money. These models, developed by Alibaba, come in various sizes, including some super-efficient 'Mixture of Experts' (MoE) versions and more traditional 'dense' models, offering strong performance, especially in coding and agent tasks.
Here's a breakdown of what makes Qwen3 stand out:
Benchmarking & Performance:
- Qwen3 235B (Flagship MoE Model):
- This is a big model with 235 billion total parameters, but only 22 billion are 'active' at any time, making it potentially more efficient than its size suggests.
- It goes head-to-head with top models like Gemini 2.5 Pro, O1, DeepSeek R1, and Grok 3 Beta.
- On Arena Hard (a benchmark measuring conversational AI), it scores very close to Gemini 2.5 Pro (92 vs 85.7).
- It slightly beats Gemini 2.5 Pro on Live Code Bench (70.7 vs 70.4) and scores higher on Code Forces ELO rating (2056 vs 2001).
- It excels in 'function calling' (letting the AI use external tools), scoring 70.8 on the BFCL benchmark compared to Gemini 2.5 Pro's 62.9.
- Qwen3 30B (Smaller MoE Model):
- This model has 30 billion total parameters but only 3 billion active ones, meaning it should be incredibly fast on capable hardware.
- It's considered potentially the best overall model in its size class.
- It even beats Gemini 2.5 Pro on the function calling benchmark (70.3 vs 62.9).
- Compared to models like Qwen 2.5, Gemma 3 27B, Deep Seek V3, and older GPT-4.0, it shows significant improvements across benchmarks like Arena Hard, Amy 24/25, and Live Code Bench.
- Comparison to Llama 4:
- Qwen3 235B generally outperforms Llama 4 Maverick (a 402B parameter model) across most standard benchmarks like MMLU, GPQA, and GSM8K, despite Llama 4 having more total parameters.
- This release is seen as potentially overshadowing Llama 4, especially given its timing just before LlamaCon.
- Independent Benchmarks (Artificial Analysis):
- On the GPQA Diamond benchmark (testing scientific reasoning), the flagship Qwen3 235B model scores 70%.
- While still behind Gemini 2.5 Pro (84%) and O3 (near 84%), it performs well, landing just behind DeepSeek R1 and Llama 3.1 Nemetron Ultra.
- The smaller Qwen3 30B MoE model shows remarkable efficiency, scoring well on GPQA Diamond relative to its very small number of active parameters (3B).
Unique Features:
- Hybrid Thinking Model:
- This is a really cool feature not commonly seen.
- Models can operate in 'Thinking Mode' (taking time to reason step-by-step for complex tasks) or 'Non-thinking Mode' (providing quick answers for simpler requests).
- Crucially, you can adjust the 'thinking budget' – how many computational tokens it uses for thinking.
- More thinking tokens generally lead to better performance on harder tasks, allowing a trade-off between speed/cost and quality.
- This is great for tasks like coding where sometimes you need deep thought (building a feature) and sometimes just quick execution (running commands).
- Tool Calling During Chain of Thought (CoT):
- This is another advanced feature, previously mostly seen in OpenAI's models (O3/O4).
- The model can think, decide it needs a tool (like fetching data or using a code interpreter), use the tool, get the result, and then continue thinking within the same reasoning process, all without needing a new prompt.
- Demos showed it successfully fetching GitHub stars, plotting charts, and organizing desktop files by type, seamlessly integrating thinking and tool use.
Model Details & Training:
- Model Family: Two MoE models (235B/22B active, 30B/3B active) and six dense models ranging from 32B down to 600M parameters.
- Context Length: 128k tokens for the larger models (8B and up), 32k for the smaller ones (down to 600M). This is considered standard but not groundbreaking.
- Pre-training Data: Trained on a massive 36 trillion tokens (nearly double Qwen 2.5), including data from 119 languages, web data, and 'PDF-like' documents.
- Synthetic Data: Significantly used synthetic data generated by previous Qwen models (Qwen 2.5 VL, Qwen 2.5 Math, Qwen 2.5 Coder) to boost math, code, and reasoning capabilities.
- Training Stages:
- Foundation: Trained on 30T+ tokens (4k context) for basic skills.
- Knowledge Boost: Added 5T tokens focused on STEM, coding, reasoning.
- Long Context: Extended context to 32k using high-quality long-context data.
- Post-training (for Hybrid Model):
- Long Chain of Thought: Trained on complex reasoning tasks.
- Reasoning Reinforcement Learning: Used rule-based rewards to improve reasoning.
- Thinking Model Fusion: Combined long CoT data with instruction tuning data (generated by the model itself) to integrate both thinking and non-thinking modes.
- General Reinforcement Learning: Fine-tuned on general tasks to improve overall capability and safety.
- Distillation: Used 'strong-to-weak' distillation to create the smaller, high-performing dense models from the larger ones.
Availability & Testing:
- The models are open source and readily available for download.
- They can be run using tools like LM Studio (presenter is an investor), Ollama, MLX, Lama CPP, and K transformers.
- The presenter tested the 30B MoE model on a powerful Mac Studio and found it 'blazing fast' for a simple coding task (writing Snake in Python).
Overall, Qwen3 looks like a fantastic and highly capable open-source model family, bringing top-tier performance and innovative features like adjustable thinking and integrated tool use to the broader community. The presenter is clearly excited about its potential, especially for agentic tasks and coding.