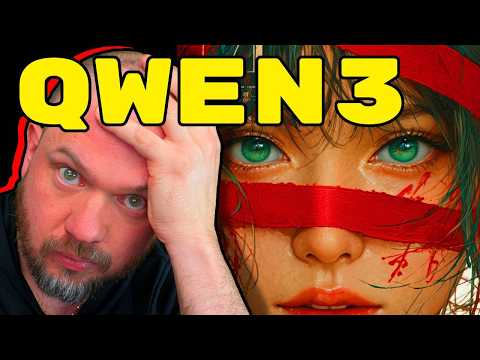
QWEN3 just BROKE the AI Industry...
Channel: Wes RothPublished: April 29th, 2025AI Score: 100
19.9K79013414:44
AI Generated Summary
Airdroplet AI v0.2A new major open-source AI model, Qwen3, just dropped unexpectedly from China, shaking things up because it's surprisingly competitive with top-tier models like Gemini 2.5 Pro and OpenAI's GPT-3.5 Mini (referred to as O3 Mini in the transcript). This release includes not just a large flagship model but also several smaller, open-weighted versions, all aimed at pushing AI research and development forward globally.
Here's a breakdown of what makes Qwen3 interesting:
- Confusing Names: Get ready for some wild naming conventions. The main model is the Qwen3-235B-A22B. Qwen3 is the model family, 235B means 235 billion total parameters (the model's 'size'), but it uses MoE (Mixture of Experts).
- Mixture of Experts (MoE) Explained: Instead of one giant brain processing everything, MoE uses specialized 'experts' within the model. Only the relevant experts (about 22 billion parameters, the 'A22B' part) activate for a given task, making it more efficient than using all 235 billion parameters every time. The opposite of MoE is a 'dense' model, which is just one big block.
- Performance Check: Qwen3 holds its own against the big players. On benchmarks like Arena Hard, AIME math competitions, Live Code Bench, and Code Forces, it often sits right between Gemini 2.5 Pro and O3 Mini, sometimes even beating them, especially in coding tasks. Keep in mind, benchmarks aren't everything – sometimes developers 'game' them, but it shows Qwen3 is seriously capable.
- Open Source Goodness: Alongside the big MoE model, they've released six 'dense' models (ranging from 32B down to 0.6B parameters) with open weights. You can find them on platforms like Hugging Face. The goal is explicitly to help everyone – researchers, developers, companies – advance AI. This open approach is seen as fantastic for global progress.
- Hidden Tricks?: One of the developers hinted that Qwen3 has some cool features not mentioned in the official documents, which could lead to new research or products. We'll have to wait and see what emerges as people experiment.
- Thinking vs. Non-Thinking Modes: A key feature is the ability to switch between a 'thinking' mode (like a reasoning model that takes time and more processing power to work through problems) and a 'non-thinking' mode (for quick, instant answers). The model is smart about managing this, using more 'thought' for hard problems and less for easy ones. Performance significantly improves on complex tasks when you let it 'think' more (use more tokens).
- Multilingual Champ: It supports a whopping 119 languages and dialects.
- Agent Capabilities: It's better at coding and acting like an 'agent' (performing tasks using tools). It even supports Anthropic's protocol (MCP) for interacting with software.
- Massive Training Data: Qwen3 was trained on nearly double the data of its predecessor (Qwen 2.5), using almost 35 trillion tokens from the web and documents. Interestingly, they used older Qwen models to help gather, filter, and improve this data, and even generate synthetic math and code data. This shows how newer AI models help build even better future models – a cycle of improvement.
- Pre-training Deep Dive: Training happened in stages:
- Basic skills from 30T+ tokens (4k context).
- Focus on knowledge (STEM, code, reasoning) with another 5T+ tokens.
- Adding high-quality long-context data to extend the context window to 32,000 tokens.
- Post-training Polish: After the initial training, the big models went through more refinement:
- Long Chain-of-Thought Cold Start: Teaching it how to reason step-by-step.
- Reasoning Reinforcement Learning (RL): Rewarding it for getting correct answers through reasoning.
- Thinking Mode Fusion: Blending the thinking and non-thinking modes smoothly.
- General RL: Improving instruction following, formatting, and agent skills.
- Training Smaller Models (Distillation): The smaller, lightweight models were trained using a 'strong-to-weak distillation' method. Essentially, the big models act as 'teachers,' generating high-quality examples that the smaller 'student' models learn from. This creates fast, cheap models that retain much of the capability, suitable for phones or edge devices.
- Reinforcement Learning Approach: They used RL techniques focusing on exploration (trying new things) and exploitation (using what works). It seems they didn't use DeepSeek's specific GRPO technique, which is known for being efficient by skipping a 'critic' step in RL. This difference is noted as interesting.
- Transparency and Sharing: A big plus is how openly they're sharing their methods through blog posts and an upcoming paper. This allows others to replicate their work and build upon it, accelerating innovation.
- The Future is Agents: The team believes the focus in AI is shifting from just training models to training agents that can perform tasks. They promise their next steps will bring significant advancements.
- License to Build: It uses the Apache 2.0 license, which is very permissive. You can use it commercially, modify it, build products on top of it, and sell them, as long as you give credit. This encourages businesses to adopt and innovate with Qwen3.